Sustained Economic Growth Comes From Relentless Technological Progress
Tuesday, July 16, 2024
Daniel Susskind writes in the FT urging the new UK government to invest in science and technology and the people that drive their development in order to deliver the economic growth that Keir Starmer’s government promises. He writes the following of the mechanisms of growth:
[T]he little we do know suggests that it does not actually come from the world of tangible things, but rather from the world of intangible ideas…. Or, more simply, sustained economic growth comes from relentless technological progress.
Too often governments focus on the trappings of economic growth — tangible things like housing and roads — but fail to invest adequately in the furnace that drives it: the discovery and deployment of useful ideas.
He rightly points out that leaders often have little control over the actual drivers of economic growth, certainly not within short terms that they occupy office. But if the furnace of economic productivity is fed, and the pipeline from that furnace to deployment of technologies is properly maintained, then tangibles like faster trains and better roads are the result. Productivity rises, as well as quality of life. Just not on the timescale of an election cycle.
I partly quibble with Susskind’s characterization that there is no lever for economic growth. The levers are investment in basic research, careful nurturing of promising technologies, and efficient deployment of the most promising of those. The process is not simple, nor a fast-acting one. Technological progress compounds, but only so long as governments make regular investment and smart choices.
I also want to nitpick the notion that new ideas will come less from humans and more from technology. On this Susskind writes:
The current century will be different. New ideas will come less frequently from us and more from the technologies around us. We can already catch a glimpse of what lies ahead: from large companies like Google DeepMind using AlphaFold to solve the protein-folding problem to each of us at our desks using generative AI — from GPT to Dall-E.
Humans are still the agents of technologies like AlphaFold and generative AI. They choose the questions to ask and direct the process. Even if these products do begin formulating meaningful scientific questions on their own, humans will still choose which questions to pursue based on what they perceive as having the most value; the technologies are not autonomously identifying and solving problems. And when they start doing so, it will be for the benefit and with the consult of humans.
The Full Stack
Friday, May 24, 2024
The modern economy runs on scientific, engineering, and manufacturing progress. J. Bradford DeLong, in his grand narrative Slouching Towards Utopia, describes modern history as being driven by economics. The modern era was brought into existence by the development of the modern corporation, globalization, and the industrial laboratory. All of these components are necessary. Michael Strevens paints a similar picture in portions of his book, The Knowledge Machine.
What many miss is the importance of nurturing all three of these ingredients. Politicians and administrators want the niceties that the confluence of these institutions brings and they want them now. They focus on the institutions closest to the output end of the pipeline, the part where the emerging product is becoming visible. The exciting part. Money then gets funneled to the last mile of the stack while input is neglected. This is a mistake. I will argue why governments must consider the full stack and not lose sight of the slower moving, but perhaps even more crucial, elements of the pipeline.
To mix metaphors, the technological stack can be thought of as a house. Basic science is the foundation. Here lies the forefront of human knowledge. It is the cutting edge. How the technological foundation is built will determine how the rest of the building can be constructed. This means choosing the right questions to pursue with a balance of scattershot, high-risk high-reward type research and clear pathways to practical technologies. The goal is to surface interesting questions that will open avenues that contain yet more interesting questions. From there applied science and engineering science take some of that basic knowledge and explore how it might be made into useful technologies. After applied science is the engineering phase. Ideas are worked until they can be made useful give the wants and needs of society.
Manufacturing is the next layer of the stack. By this I do not merely mean making the thing. Manufacturing is itself a complicated globe-spanning pipeline that includes procurement of raw materials and processing of those materials. Components fashioned are then shipped to hubs for final assembly. The process is an engineering effort that leads to economies of scale to make products affordable to consumers. Simply engineering a new product is not enough if producing it is expensive. An entirely different kind of engineer is required to scale an idea so that economies of scale can work its magic to successfully bring a product to market.
My focus is on the under-funded, slow moving bottom of the stack: basic science. Practitioners of basic science are often poor marketers of their craft. They often resent the market economy and do not recognize their place within it. They see themselves as outside of it. When describing the importance of their profession they talk in romantic terms, as being “passionate” and describing the innate curiosity of humankind as the driving force. This is all well and good (and is indeed the reason that I am a scientist) but another sense of “greater good” is the economic one: building the furnace of the economic engine that brings down the price of goods, raises standards of living, delivers medicines. Science is the bedrock of this economic movement that has been responsible for the enormous reduction in poverty over the long twentieth century. The sooner scientists recognize our role within the economic engine instead of resenting it, the better we can make the case for significant increases in basic research spending.
Now that I have obtained my Ph.D. and have achieved some stability as a working scientist, this topic will become a focus. What can be done to reverse the trend of declining spending on basic research? How can we increase the appetite for risk and take more chances on promising technologies? These and other questions will be explored in these virtual pages.
More Podcast Paywalls
Tuesday, October 31, 2023
The Economist has moved all of its podcasts behind a paywall except for its daily news show The Intelligence. This is disappointing because I somewhat regularly would share episodes with friends who don’t subscribe to the newspaper. It also means that the analogy of podcasts being like radio that you download no longer holds. Another idea recedes into the past. I get the move — the advertising market is drying up and many independent podcasts are moving toward membership models. Spotify has pushed the industry towards subscription and now Apple Podcasts has gotton on board. Still, if The Economist is going to move its content behind a paywall, at least they have done it the right way. You can still use any podcast player to listen to shows. They provide a subscriber RSS feed in addition to hooking into the subscriber features of the big podcast apps. This is definitely the way to go and I’m glad they are continuing to use RSS instead of making up their own to do it.
Previously I posted about The New York Times launching its own audio app. I still think this is doomed to fail. They have since launched more shows that are available only on the app. I don’t know their numbers, but I suspect they won’t see a lot of growth in the long run. Thinking big picture, the internet is now going through a phase of decentralization. This is most apparent in the social media space with the rise of new microblogging platforms like Mastodon and Threads — and more importantly ActivityPub which allows them all to interconnect — and the slowly disintegrating Twitter/X. Podcasts have always used use-it-anywhere RSS feeds and I don’t see that changing any time soon.
Apple Silicon Macs have a DAC that supports high-impedance headphones
Friday, June 23, 2023
I bought the Blue Mo-Fi headphones shortly after they came out in 2014. They great headphones, but the fake leather on the ear pads have almost completely flaked off and now that Blue has been bought by Logitech and is killing the Blue mic brand there is little hope of trying to get replacement parts or repair them in the future (I have tried). Besides, they didn’t have stellar reviews when they came out and now I’m getting into high-fidelity audio.
Wading through the online world of audiophile hardware was making me consider buying a DAC and amp in addition to new headphones, but then I found Apple’s Support pages for lossless audio and it appears that Apple Silicon Macs not only support lossless audio output, but also have a built-in DAC and amp that can drive high-impedance headphones. That solves that problem. I’ll just buy some entry-level audiophile headphones and go. The built-in hardware is probably not as sophisticated as dedicated hardware, but I doubt I’ll ever be that into the highest-end audio equipment. There are other things to be obsessed about.
I can’t find any information on the built-in DAC or amplifier in System Information, but the Audio MIDI Setup app (comes with macOS) allows you to select the input and output sample rate and other settings.
Links to Apple’s support pages:
About lossless audio in Apple Music
“The entire Apple Music catalog is encoded in ALAC in resolutions ranging from 16-bit/44.1 kHz (CD Quality) up to 24-bit/192 kHz.”
Supported on iPhone, iPad, Mac, HomePod, Apple TV 4K (not greater than 48 kHz), and Android.
This page says only the 14-inch and 16-inch MacBook Pros support native playback up to 96 kHz, but I think this is outdated because the other support pages all say otherwise.
Use high-impedance headphones with your Mac
Macs introduced in 2021 or later (probably meaning M1 chips or later).
Impedance detection and adaptive voltage output, and built-in digital-to-analog converter (DAC) that supports sample rates up to 96 kHz.
Play high sample rate audio on your Mac
Hardware digital-to-analog converter (DAC) that supports sample rates up to 96 kHz in Macs introduced in 2021 or later (i.e. M1 chips or later).
Set up audio devices on Audio MIDI Setup on Mac
“To set the sample rate for the headphone jack, use the Audio Midi Setup app, which is located in the Utilities folder of your Applications folder. Make sure to connect your device to the headphone jack. In the sidebar of Audio MIDI Setup, select External Headphones, then choose a sample rate from the Format pop-up menu. For best results, match the sample rate for the headphone jack with the sample rate of your source material.”
Some recent Reddit threads on the topic:
- MacBook Pro as a AMP & DAC
- External DAC for Mac vs. built-in Mac DAC
- New MacBook Pro M1 Built-in DAC Rocks!
- Does a DAC make sense on Mac mini M2?
The New York Times Makes a Podcast-like App
Tuesday, May 23, 2023
The New York Times just released New York Times Audio, an app for “audio journalism”. It curates all of the New York Times podcasts (including a new daily podcast called “Headlines”) as well as podcasts from third parties, like Foreign Policy and This American Life. It will also include audio versions of written articles.
I think it will be difficult to penetrate the pretty well established spoken-word market. Podcasts are dominated by Apple Podcasts, and Spotify has had a hard time turning podcasting into a core part of its business. I can see NYT Audio being a niche product that appeals to a small subset of NYT subscribers, but not much more. I’m guessing the goal is to charge a fee for third parties to access NYT subscribers. I don’t really see how this app would generate more revenue from existing subscribers both because I don’t see huge numbers using the app and because podcasts are traditionally free and use open web standards. Again, see Spotify’s and other attempts to make proprietary podcasting formats.
I’ll try the app, but I don’t see it becoming a habit. Overcast is already on my Home Screen and adding another podcast app is a tall order. If I find something I like, I will most likely just add it to a playlist in Overcast.
Plastics Are Almost All Downside
Thursday, December 29, 2022
This piece by Judith Enck, a former EPA regional administrator, and Jan Dell, a chemical engineer, in the Atlantic highlight three main problems with plastic recycling.
- The large number of types of plastics make sorting and recycling difficult.
Just one fast-food meal can involve many different types of single-use plastic, including PET#1, HDPE#2, LDPE#4, PP#5, and PS#6 cups, lids, clamshells, trays, bags, and cutlery, which cannot be recycled together.
- Processing plastic waste is toxic and wasteful.
Unlike metal and glass, plastics are not inert. Plastic products can include toxic additives and absorb chemicals, and are generally collected in curbside bins filled with possibly dangerous materials such as plastic pesticide containers. According to a report published by the Canadian government, toxicity risks in recycled plastic prohibit “the vast majority of plastic products and packaging produced” from being recycled into food-grade packaging.
- Recycling plastic is not economical.
Yet another problem is that plastic recycling is simply not economical. Recycled plastic costs more than new plastic because collecting, sorting, transporting, and reprocessing plastic waste is exorbitantly expensive. The petrochemical industry is rapidly expanding, which will further lower the cost of new plastic.
In addition, there is a growing body of evidence showing that plastics break down into microplastics that permeate the environment, and humans and animals end up ingesting them. There are microplastics in all corners of the earth and researchers have been trying to understand their effects on human health.
This is frankly alarming, and I’ve been more and more shocked every year since the plastics issue has been making it into the mainstream press. I’ve been cutting down on the amount of plastic goods I purchased and several years ago I stopped storing food in plastic containers, opting for metal or glass. Last year, I stopped buying clothing made from synthetic materials (as much as I can), since clothing releases a huge amount of microplastics into the water supply in every wash. Now I buy cotton, wool, and linen clothing almost exclusively. I find myself paying more attention to the materials of pretty much every product I plan to purchase. It definitely feels like an uphill battle because of the sheer amount of plastic that is reported to be in our surroundings.
Plastic Recycling Doesn’t Work and Will Never Work
Classrooms will need to change with generative AI
Friday, December 16, 2022
Zeynep Tufekci, in an op-ed for the New York Times, ponders the implications of generative AI as we very likely enter the dawn of a new technological era.
Teachers could assign a complicated topic and allow students to use such tools as part of their research. Assessing the veracity and reliability of these A.I.-generated notes and using them to create an essay would be done in the classroom, with guidance and instruction from teachers. The goal would be to increase the quality and the complexity of the argument.
This would require more teachers to provide detailed feedback. Unless sufficient resources are provided equitably, adapting to conversational A.I. in flipped classrooms could exacerbate inequalities.
In schools with fewer resources, some students may end up turning in A.I.-produced essays without obtaining useful skills or really knowing what they have written.
I 100% agree. The coming sophisticated AI will demand that schools use more labor-intensive teaching methods. She is also right that this will exacerbate inequalities unless we as a society put a lot more money into our education systems.
What Would Plato Say About ChatGPT?
Introducing TransferMatrix.jl
Friday, November 04, 2022
I’m excited to introduce my first software package written for broad use. TransferMatrix.jl is a general 4 x 4 transfer matrix implementation written in the Julia programming language. The transfer matrix method analyzes the propagation of an electromagnetic wave through a multi-layered medium. You can compute the reflectance and transmittance spectra, as well as calculate the electric field profile as a function of position within the medium.
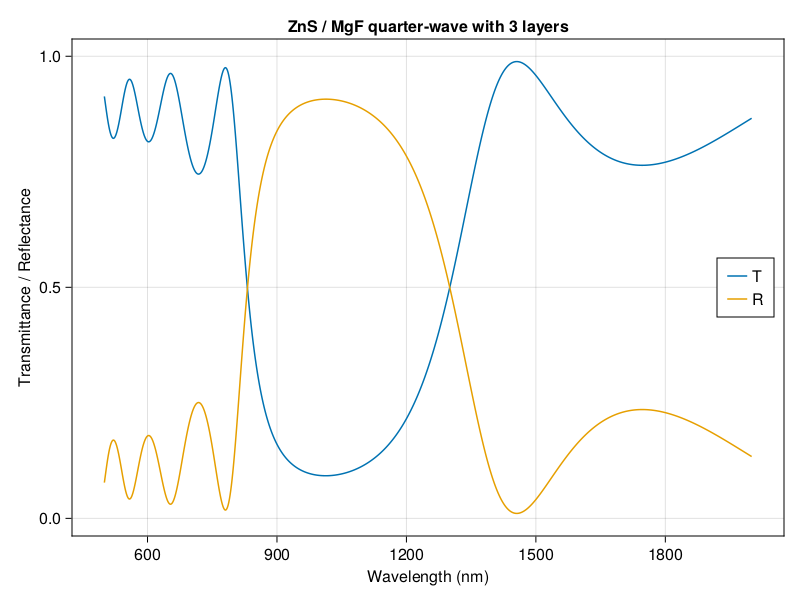
I started with some simple code in Python for my own projects and sharing it with other in my lab, but it had some limitations and I was growing to love coding in Julia. I didn’t want to switch to Python to do just this one thing. I started rewriting the code in Julia on the weekends, but I didn’t just want to reimplement what I had done in Python. You see, I’ve found that there are a lot of transfer matrix implementations on the web. It seems like every grad student doing something in optics or thin films writes one, plops it on the web, and lets it get stale when they graduate. A simple 2 x 2 algorithm is not hard to write but it can’t be fully generalized. I was also frustrated that there are all of these papers that try to improve the method (transfer matrices, apparently, are still an active area of research), but the code is difficult to read, poorly documented, untested, used poor programming practices, and abandonded.
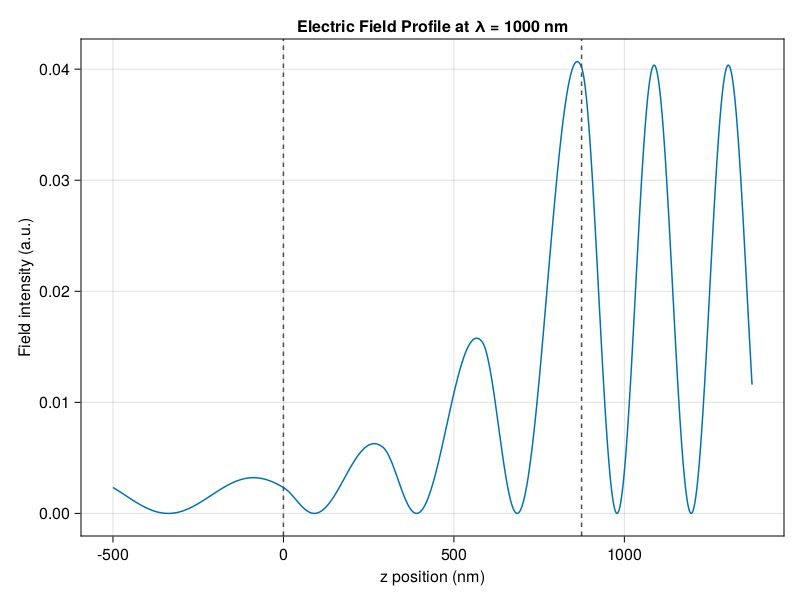
I wanted to write something based on the latest developments that dealt with the shortfalls of the traditional transfer matrix (singularities and numerical instabilities), while being highly modular, reusable, and with great documentation and tutorials. And I wanted it in Julia to take advantage of Julia’s speed and the scientific community over there.
High modularity means that each function is as small as it can be. This makes it easy for someone to replace one or more steps with something custom to test a new idea and improve on the method in their own research. It means that it is easy to test and easy to read the code (in pure Julia).
Julia’s package manager makes it easy to install. Everything is documented and I have written an extensive tutorial — all of the code in the tutorial can be run as is.
Sharing and reuse is easy. You can make a config file with all of the simulation parameters (even the refractive index data from a file) and reproduce the results for that structure. You can create multiple variations easily this way and share the exact configuration that you used with others. Even complicated periodic structures are easy to make this way.
This implementation is based on the latest research in general transfer matrix methods and every piece of research that I use is cited at the function level, complete with the DOI so that you can follow everything that has been done and make precise modifications. A full list of references is also on the documentation website.
My hope is for this to be at least a first stop for someone looking for a transfer matrix algorithm. If the community likes it, then I would like this to become a part of a standard set of science or physics packages that currently exist in the Julia ecosystem. Ease of use and readability really were my priorities — there is little boilerplate code. And Julia’s speed means you can do wavelength and angle-tuning simulations to produce 2D contour plots quickly. Together with the generality of this implementation based on current research, I hope that others can use TransferMatrix.jl to try out new ideas.
Try it out and please leave feedback!
More on sewage monitoring
Tuesday, November 01, 2022
Sewage monitoring is low-level persistent in the news. Former members of President Biden’s Covid-19 advisory board write in the New York Times
the national reporting system for collecting and testing samples from wastewater treatment systems for Covid remains limited, uncoordinated and insufficiently standardized for a robust national surveillance system. If public health officials can’t track the data to mobilize a response to a crisis, the information that has been collected doesn’t do much good.
I had thought a program like this would have made it into the recently-passed infrastructure package. It would be a shame if health and safety monitoring systems (like wildfire or region-wide earthquake monitoring) were not built or strengthened in the near future.
Here’s more details on sewage on Jim Al-Khalili’s excellent BBC podcast, The Life Scientific:
Jumping over the time-to-first-plot problem in Julia
Wednesday, October 26, 2022
I’ve been using Julia for about a year now after moving my entire workflow from Python. When I sometimes revisit Python I am so glad I made the switch. No regrets whatsoever. Julia still has one pain point, which is time to first execution (TTFX) or time to first plot (TTFP)1. But even this “pain point” is somewhat bizarre because Julia is a compiled language. Of course there is going to be a compilation step that will make it slow to get going. What makes this a pain point is the desire to have it all — “we are greedy,” say the founders of the language. Julia wants to be interactive and dynamic, but compiled and fast. But the fact that it’s compiled means that when a user wants to make a simple line plot it takes two minutes to precompile the plotting library, compile the plotting functions, and finally show the plot on screen. Only after that initial setup are all subsequent plots instant — as long as you keep your session active. There are many more talented programmers in the community than me, and one user in a recent Discourse thread explained the tradeoff and the difficulty in reducing compile time:
A tangent: I believe it is worthwhile to discuss why this is such a phenomenally big problem in julia. Julia has two very special features other languages do not share: (1) multimethods as the fundamental principle for the entirety of the ecosystem and (2) compiled code. It is very difficult to know what code you need compiled and to not discard the vast majority of already compiled code when importing new libraries that add new methods for pre-existing functions. No one has had to deal with this problem before julia. It is being slowly dealt with. Sysimages basically carry the promise that no significant amount of new methods will be defined, hence they can cache more compiled code (this is very oversimplified borderline misleading explanation).
That last point about sysimages is interesting. Making a sysimage in Visual Studio Code is a big workflow improvement, and I recommend all Julia users try it. It essentially compiles all the libraries from your project, and any other files you specify, and puts them into a file. I guess you could say it freezes your Julia session to use later. This is faster than precompiling each time. It’s built into the Julia extension and easy to set up. Detailed instructions are on the Julia VS Code extension website, but in a nutshell the steps are:
- Open your project folder in VS Code with the Julia extension installed (and make sure it’s activated)
- Make a new folder called
.vscode
- Make a file called
JuliaSysimage.toml
in that folder - Paste the
[sysimage]
text below this list into that file - Select
Tasks: Run Build Task
and thenselect Julia: Build custom sysimage for current environment
- Check the
useCustomSysimage
setting in the Julia extension settings in VS Code - Restart the Julia REPL. (Hit the trash can button and open a new REPL session from the Command Palette)
Copy and paste this into a JuliaSysimage.toml
file:
[sysimage]
exclude=[] # Additional packages to be exlucded in the system image
statements_files=[] # Precompile statements files to be used, relative to the project folder
execution_files=[] # Precompile execution files to be used, relative to the project folder
The extension automatically uses the sysimage instead of precompiling your project. And now your project should run much faster and TTFX will be significantly sped-up. On my M1 iMac I use the powerful but compiler-heavy Makie plotting library and I went from waiting about 2 minutes for precompilation and maybe 30 seconds for that first plot to almost no compile time, and execution in less than a second. (Other people have properly benchmarked this, I’m not going to do that here). I see similar results on my 2019 Intel Macbook Pro.
But here’s what really got my workflow sailing. I’m PhD student working in experimental physics. I have a lot of messy data and I need to make a ton of plots to explore that data. I have a top-level folder for my experiment.
In there I have separate folders for raw data, daily scripts, and results/plots.
Then I have a src
folder where plotting, analysis, and file reading/writing scripts go. The files in src
rarely change, so that means I can add them to the execution_files
section in my JuliaSysimage.toml
file. These scripts get compiled along with all my plotting packages into the sysimage. This makes everything fast.
My Experiment Folder
|
|_.vscode
| |_JuliaSysimage.toml
|_data
| |_221015
| |_221025
| |_...
|_lab_notebook
| |_221015.jl # julia scripts for that experiment day
| |_221025.jl
|_results
| |_plots
| |_221015
| | |_fig1.pdf
| |_...
|
|_src
| |_plotting_functions.jl
| |_data_io.jl
| |_lab_notebook_template.jl
| |_analysis_functions.jl
|
|_JuliaSysimage.dylib
|_Project.toml
|_Manifest.toml
|_...
For the above example, I would add the files
execution_files=["src/plotting_functions.jl", "src/data_io.jl", "src/analysis_functions.jl"]
to JuliaSysimage.toml
. As long as I don’t change these files, their functions load instantly. The functions in these files are used in my lab_notebook
files with an include()
statement at the top (e.g. include("plotting_functions.jl")
). For example, I have custom plotting functions and themes that make an interactive grid of plots with toggles and settings so I can look at and compare data exactly the way I want. Recreating the sysimage a couple of times a month (or even once a week) is not a big deal compared to the time savings I get every day.
As an aside, I recommend everyone have some kind of setup like this where you reuse plotting and analysis functions, no matter what language you’re using. If you are editing these functions every day then either these scripts have not settled down yet or something isn’t quite right with the workflow. It is worth it to sit down and figure out what tools you need to build to smooth out day-to-day computational tasks instead of writing scripts from scratch each time you have to make a graph of some data. For the most part, the file format for my data is the same, so I only need a handful of plotting and data read/write functions. Once they’re written, that’s it. I can move on.
As many others have said, the time-to-first-X problem is a priority for the Julia developers. The version 1.8 update this year saw some speedups, and I think the expectation is that this will continue in future 1.x releases. These improvements to the compilation stage, both in VS Code and the work being done in the language itself, have surpassed my expectations. I thought Julia would always have an initial lag and that people would have to make hacks and workarounds. This really is exciting, and there is a lot to look forward to in Julia’s future.
-
The plotting libraries generally take the longest to precompile. ↩
The Structures of the Scientific Enterprise
Monday, October 17, 2022
Geoff Anders of Leverage Research, a non-profit that writes scientific papers without publishing them in peer-reviewed journals (so it seems), writes in Palladium Magazine a brief summary of the role of science through the ages. His overall theme is clear from the section headers. He sees science as going from largely an endeavor of wealthy individuals to one that obtained authority from the state. I quibble with parts of Anders’s historical narrative, but there are some good ideas in his conclusion.
I’m not sure how big of a phase “science as a public phenomenon” was. He makes it seems like science was a circus show in the 16th, 17th, and parts of the 18th century. I think this an exaggeration, but I’m not a science historian (and neither is he). Anders also relies too heavily on a single instance of science being used authoritatively (King Louis XVI’s commission to investigate Franz Mesmer’s methods of apparent hypnosis) to make the case that science had become broadly authoritative. This strikes me as a weak way to make the argument. I would be hesitant to say science has ever had the authority he seems to imply that it had. A massive influence? Definitely. A justification? Probably, and sometimes a scapegoat. But I wouldn’t call it an “authority”. I think if one were to use that word it needs a bit more context, which Anders does not provide.
His section on science and the state overemphasizes military technologies and glosses over quality of life improvements that raised large parts of the global population out of abject poverty (see Bradford DeLong’s excellent grand narrative, Slouching Towards Utopia published recently).
The one area that I think Anders has something going for him is his conclusion. The scientific community is at some sort of crossroads in terms of funding and elements of how it is structured. I see a lot of complaints that funding for blue-sky ideas is drying up and there are reports that hiring is becoming difficult. Some of this likely has to do with how universities are funded — and that is a whole other can of worms.
I like Anders’s idea of splitting science into two camps: exploratory science and settled science. At first glance one might say that science is exploratory and that the settled part is taken care of by applied scientists and engineers, but I think Anders’s argument is more subtle. He says that funding might be restructured so that exploratory science is decentralized and career tracks are split into “later-state” and “earlier-stage” science. I would take this idea further. First, by establishing an exploratory wing of science its mission will be to take big risks with the expectation of failure — and failures should be reported and praised. This wing would be analogous to the US’s DARPA initiative.
Second, a later-stage wing of science wouldn’t have to feign novelty where none exists. They would be free to solidify existing science1. Maybe they can bundle a few studies from the exploratory stage and make that science robust, ready to pass the baton to the applied scientists and engineers.
I think the separation into early-phase and late-phase science would be a boon for the scientific endeavor. It would strengthen the pipeline from basic science to societal improvements. It would also clarify the mission of any given scientific project. Having a later-stage project would carry just as much importance as an exploratory project within its domain, and exploratory labs would be free to try out pie-in-the-sky ideas without fear of blowback from funding agencies.
-
I’m not sure how this has to do with Anders’s idea of “don’t trust the science”. I think he is throwing a bunch of ideas together without a clear thread (what does decentralization get you besides being hip with the crypto crowd?), but there are some nuggets in here worth thinking about. ↩
Sewage Monitoring
Saturday, October 08, 2022
I have seen a few articles in the press this year on sewage monitoring for tracking disease and the health of a city. Sara Reardon writing for Scientific American reports on how wastewater monitoring has been taken up as a tool by the CDC and local communities for tracking COVID and other diseases in the US. The impact of wastewater data aggregation and analysis could be huge — in both the positive and negative. It strikes me that governments are largely reactionary to changes in public health. Little attention is paid to preventative measures. This could change that.
Thinking more broadly, I think this tool has much greater potential than disease tracking. Combining wastewater data with other inputs could be a monumental shift in understanding the health of a community on quite a granular level — both in terms of what substances are circulating in a community and the potential for real-time fidelity. You can imagine wastewater data being combined with data from hospitals, air quality monitoring, or even news of major events affecting the mental health of a city.
A recent article in The Economist gives a broad perspective on wastewater monitoring and how it is being used globally, including the benefits and potential dangers of sewage data collection.
Quasiparticles and What is "Real"
Friday, October 07, 2022
This is a bit of an old post, but one that I liked a lot considering that I am also in the business of manufacturing quasiparticles (mine are polaritons). It’s fascinating that the quasiparticles that appear because of material excitations can be described using many of the same models as “real” elementary particles.
Read the post on nanoscale views by Douglas Natelson.
Martin Rees: How Science Can Save the World
Thursday, September 29, 2022
Martin Rees talks to The Economist’s Alok Jha on existential risks to civilization and the importance of science and science communication in the 21st century running up to his new book coming out this November (I already pre-ordered).
There is a constant buzz on Twitter about the pains of academic research. Martin Rees agrees that aspects of university research needs to be changed. Administrative bloat and scientists staying in their positions past retirement age discourage blue-sky research and gum up the promotion pipeline. He criticises the scope of UK ARIA (Advanced Research and Invention Agency) program, which is supposed to function similar to the US’s high-risk high-reward DARPA (Defence Advanced Research Projects Agency) program:
In that perspective, it’s just a sideshow. The ministers say this is a wonderful way in which scientists can work in a long-term way on blue skies research without too much administrative hassle. They’d be doing far more good if they reduced the amount of such administrative hassle in those who are supported by UKRI, which is supporting fifty times as much research as ARIA will ever do.
Science in the last ten years or so, I feel, has really gotten bogged down. I agree that blue-sky thinking has sort of gone out of fashion. How much this is a function of perverse publishing incentives, administrative hurdles, or the constant firehose of publications to keep up with, I don’t know. I’m glad a prominent and highly respected figure in the science community is calling out the inefficiencies and problems in the way science is practiced.
Listen on The Economist website: How science can save the world
Listen on Overcast podcast app.
Webb Space Telescope Images
Tuesday, September 27, 2022
The modern news cycle is a periodic deluge. I don’t get the sense that the James Webb Space Telescope launch has hit the public in the same way that the Hubble did. It seems like everyone moved on pretty quickly. I can’t help but keep going back to the Webb images and looking at them in awe, with a much better viewing experience on modern computer displays unavailable to those seeing the Hubble images for the first time.
View Webb Space Telescope images
The Future of Software Development in Science
Thursday, August 19, 2021
The future of scientific software development will be cloud-based together with apps that use web technologies rather than platform-specific (“native”) applications despite recent mobile computing hardware advances. Advancements in computing tools and languages are already changing science to, for example, improve reproducibility of results and facilitate better collaboration. These same tools are helping to move development itself into the cloud and are migrating the community to web-based technologies and away from native apps and frameworks.
Mobile development for the scientific community now means programming on a laptop since there are very few scientific tools available on tablets and phones. “Mobile” in the everyday sense refers to, of course, smartphones and tablets. Eventually, scientific programming will move to these mobile platforms. I’m thinking of a tablet that can perform analysis, run a notebook environment, or even run certain kinds of simulations. You will be able to hook it up to measurement devices1 or controllers. At conferences, you will be able to answer questions by running your actual simulation live with different variables and show it to someone. There is a lot of great desktop-class software, proprietary and open-source, that powers science today. None of this will be a part of the mobile future. It will all be done in the cloud and with web technologies.
The discussion around native versus web technology frameworks is already robust in programming circles2, so I approach the topic as a researcher looking for mobile and cross-platform solutions. I try to answer these two questions:
- What does software development look like in the future for science?
- How are existing cross-platform and mobile frameworks shaping the future of scientific development?
I briefly describe the problems of the current fragmented ecosystem, how that ecosystem is converging on open-source tools, and then how the emerging cloud-based computing paradigm will shape scientific computing on mobile devices.
The fragmented ecosystem
The trajectory of scientific programming is interesting because it seems to be converging on a few tools from a historically fragmented and siloed ecosystem. Chemists, for example, use their particular flavors of modeling and analysis software (like Gaussian or ORCA), and Fortran is used for much of climate science. The fragmentation makes sense because of the wide range of applications that scientific programming must serve, including modeling, analysis, visualization, and instrument control. Furthermore, scientists are often not trained in programming, leading to large gaps in ability even within a single laboratory.
These factors lead to several problems and realities within the programmatic scientific community. These include:
-
Code that is often not reusable or readable across (or within) scientific disciplines. An example of this is the graduate student who writes software for their project, which nobody knows how to modify after they leave.
-
Domain-specific applications that inhibit cross-disciplinary collaboration. This includes proprietary software that, while effective, is not shareable because of cost or underutilization. Barriers to entry exist also because only a subset of people learn how to use a particular piece of software and would-be collaborators use something different.
-
Complicated old code that stalls development. Changing an old code base is a monumental task because the expertise that created the code has moved on. This is often the case with complex and large code bases that work, but nobody knows how. Making changes or sharing can require a complete rewrite.
The problems are more apparent today because the frontiers of science are increasingly cross-disciplinary. Without shareable and reusable code, there is considerable friction when trying to collaborate3.
Convergence to open-source tools
Several technologies are now maturing and their convergence is solving some of these problems. The transition will take a long time — decades-old code bases need to be rewritten and new libraries need to be built — but I expect the scientific programming landscape to be very different ten years from now.
The wide-spread adoption of Python, R, and Jupyter in the scientific community has solved many of the readability the share-ability problems4. Many projects now bundle Jupyter notebooks to demonstrate how the code works. Python is easy to read, easy to write, and open-source, making it an obvious choice for many to replace proprietary analysis software. The interactive coding environment of Jupyter is also having a major impact on scientific coding. Someone reading a scientific paper no longer has to take the author’s word that the modeling and analysis are sound; they can go on GitHub and run the software themselves.
A level above programming languages is apps for developing scientific software and doing analysis. There are a lot of apps out there, but a major component of development will use web technologies because of their inherent interoperability. Jupyter notebooks, for example, can be opened in the browser, meaning anyone can create and share something created in Jupyter without obscure or proprietary software. Jupyter can now also be used in Visual Studio Code, the popular, flexible, and rapidly-improving editor that is based on the web-technology platform, Electron.
The growing popularity of web technologies in science foreshadows the biggest change on the horizon, the move to cloud-based computing.
Cloud-based computing for science
Mobile devices are finally powerful and flexible enough that most people’s primary computing device is a smartphone. If this is the case, then one might think that they must be powerful enough for scientific applications. So, where are all of these great tools?
Ever since the iPad Pro came out in 20185, I have been searching for ways to fit it into my research workflow. So far, the best use-case for it is reading and annotating journal articles. This great, but nowhere near the mobile computing workstation I outlined above. The reason I still cannot do analysis or share a simulation on an iPad is that Python, Jupyter, an editor, graphing software, etc. are not available for it — and my iPad is faster and more powerful (in many respects) than my Mac6.
As I look around for solutions, it seems that the answer is to wait for cloud-based development to mature. Jupyter already has notebooks in the cloud via JupyterHub. A service called Binder promises to host notebook repositories and make code “immediately reproducible by anyone, anywhere”. Github will soon debut its Codespaces cloud platform, and the Julia community (a promising open-source scientific programming language) has put their resources into Jupyter and VS Code. Julia Computing has also introduced JuliaHub, Julia’s answer to cloud computing. Legacy tools for science trying to stay relevant are also moving to the cloud (see MatLab in the cloud, Mathematica Online, etc.). Any app or platform that does not make the move will likely become irrelevant as code-bases transition.
There are no mobile-first solutions from any of the major players in scientific software despite the incredible progress in mobile hardware7. Today I can write and run my software in a first-generation cloud-based environment or switch to my traditional computing workstation.
Conclusion
What lies ahead for scientific programming? Maybe Julia will continue its meteoric trajectory and become the de facto programming language for science and scientific papers will come attached with Jupyter notebooks. Maybe code will become so easy to share and reuse that the niche and proprietary software that keeps the disciplines siloed will become obsolete. These would be huge changes for the scientific community, but I think any of these kinds of changes in the software space are compounded by the coming cloud computing shift. Scientific development will happen in the cloud and code will be more reproducible and shareable than it is today as a result.
This future is different from the mobile computing world that I imagined, where devices would shrink and simultaneously become powerful enough that a thin computing slab empowered by a suite of on-device scientific tools could fulfill most of my computing needs. Instead, the mobile device will become a window to servers that will host my software. Reproducible and reusable code will proliferate as a result, but where does that leave the raw power of mobile computing devices?
-
This just became possible with the Moku devices coming out of Liquid Instruments. ↩
-
See the current controversy over OnePassword choosing to make their macOS app using Electron instead of one of Apple’s frameworks. ↩
-
Katharine Hyatt describes these problems in the first few minutes of an excellent talk on using Julia for Quantum Physics. ↩
-
Another potential avenue for convergence is the ascent of the Julia open-source programming language, which promises to replace both high-performance code, higher-level analysis software, while making code reuse easy and natural. The language is still far from any sort of standard, but there are promising examples of its use. ↩
-
The iPad is, unfortunately, the only real contender in the mobile platform space. The Android ecosystem has not yet come up with a serious competitor that matches the performance of the iPad. ↩
-
Specifically, Apple does not allow code execution on its mobile operating systems. ↩
-
A great Twitter thread by Steven Sinofsky, former head of Windows, details the evolution of computing devices. ↩